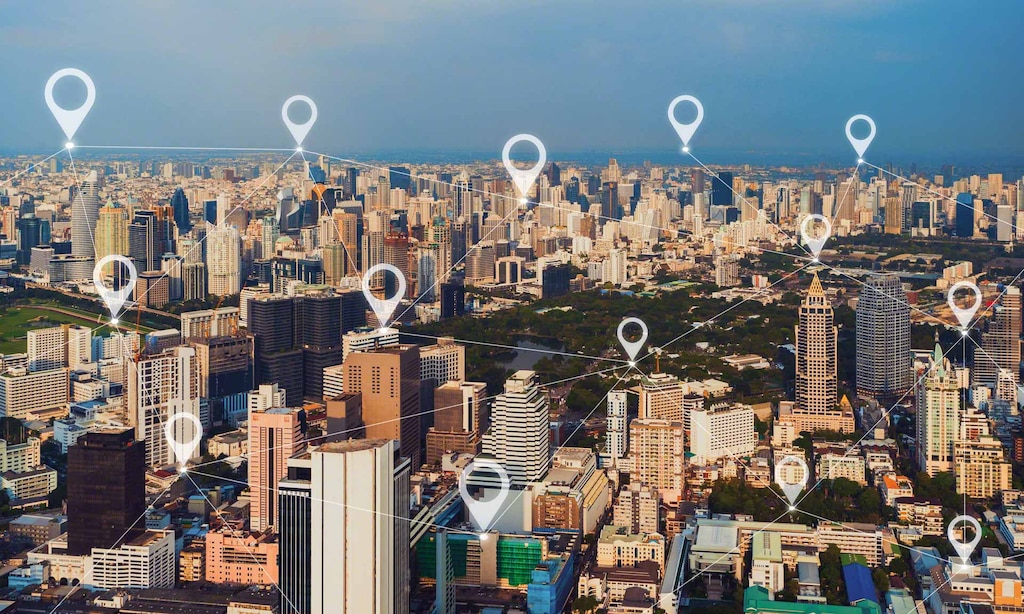
IN-DEPTH ANALYSIS
By Matthias Winkenbach
Route management is a critical part of logistics and goods transportation. It can mean the difference between success and failure in order delivery. Vehicle routing has been one of the most studied issues in logistics over the past 100 years. However, despite such attention and the major strides made, optimal efficiency in route management and last-mile logistics remains just out of reach. Leveraging the latest technological advancements in artificial intelligence (AI) and machine learning (ML) might be the key to finding the remaining pieces of this puzzle.
The foundational basis of vehicle routing is the traveling salesman problem. Academics have been studying this since the early 1800s, but the vehicle routing problem (VRP) we study today in the context of logistics was formally introduced in 1959 by the researchers George Dantzig and John Ramser. Since then, generations of researchers have studied myriads of variations and extensions of the VRP in an attempt to solve a wide range of problems in logistics and related fields. Today, there are many excellent algorithms and models to capture much of the real-world complexity inherent in real-world routing problems. Industry and academia have come much closer to mastering state-of-the-art routing problems since Dantzig and Ramser started this field 65 years ago. Nevertheless, existing methodological approaches only bring us about 80% of the way there. The remaining 20% were not as crucial in a world where people did not shop online and timely and flexible transportation options were not critical in satisfying customer needs. However, in the last couple of years, consumer expectations have increased dramatically. Customers are demanding unprecedented speed and levels of personalization. Suddenly, for the highly responsive logistics services people are asking for today, those last 20% matter a lot.
The big vans we see on the road typically make around 120 stops a day
What makes last-mile logistics particularly complex is its increasingly fragmented and constrained routes. Commuters, for instance, look for the shortest, most convenient, or fastest path from point A to point B. An Amazon or UPS delivery van, on the other hand, has to make multiple deliveries along one consolidated and efficient route. The big vans we see on the road typically make around 120 stops a day. They have to be sequenced in a way that — although the shortest path between two customers may not always be possible — the overall route is optimized. The goal is to find the fastest, shortest, most cost-effective path to connect those 120 points on the map, while respecting customer-specific constraints such as delivery time windows, parking or access restrictions, and customer availability.
This is not just a math problem, though. Last-mile efficiency is a human problem because people — for instance, drivers — are a major source of uncertainty. A driver’s experience and familiarity with a delivery region, or simply the question of whether they are having a good or a bad day, affects their ability to interact with other participants in the mobility system and to execute an efficient route. Even though companies optimize their routes as much as possible, unforeseen events such as traffic accidents can completely change the assumptions around travel times from one customer to another. For a company, many of these uncertain factors are hard if not impossible to control, and this is where traditional approaches to this problem struggle to find a solution.
Putting the customer front and center
About 10 years ago, we started doing research in this area with a focus on last-mile logistics in so-called megacities, simply because last-mile logistics processes tend to be the most complex in areas of extreme population density. Density is one of the main drivers of last-mile complexity because most cities were not planned for the size they are today. They grew over time, but their transportation infrastructure failed to expand proportionately. They were never designed to deal with the large number of people and goods that need to move in and out of them every day. As cities grow, the demand for goods and services increases along with the need for mobility of both people and goods. The result is a substantial increase in traffic load on a very hard-to-expand road infrastructure — in other words: congestion.
High demand density can have both positive and negative effects on last-mile logistics processes. With customers highly concentrated in a confined area, theoretically, couriers have to travel less to deliver the same amount of goods. Delivery routes should become more productive and hence more cost-efficient. The downside, as discussed, is a more and more congested road network on which travel times often become longer and increasingly uncertain, as even minor road network disruptions such as accidents can lead to severe traffic jams. So, while the theoretical travel distance between customers may decrease in a high-density urban environment, the total duration and hence productiveness of a route may deteriorate and become less predictable than in a low-density rural environment.
The last mile represents around 40% of total logistics costs for many companies
We are now exploring new methods inspired by AI and ML because the world is moving faster than these traditional methods can keep up. Similarly, the trend towards more customer centricity — focusing logistics processes around individual requests — makes solving modern-age state-of-the-art routing problems challenging. This is why more data-driven approaches are required. The models and algorithms we create to solve these problems need to be capable of learning and continuously updating more fine-grained information about the real characteristics of the problem. They need to develop a dynamically evolving understanding of the specific characteristics, behavior, and constraints of individual customers, particular road links, specific vehicles or drivers, and so on. This is where AI and ML methods have a unique advantage over traditional operations research (OR) approaches.
The last mile represents around 40% of total logistics costs for companies. Thus, they have to be able to predict — at a very high spatial but also temporal resolution — when and where a product will be bought. They need to know, for instance, how many items of the same product will be purchased in a specific zip code within the next 30 minutes. This is another example of how a well-established problem in the logistics industry, such as demand forecasting, is becoming reliant on state-of-the-art machine learning methods to cope with the increasingly demanding predictive capabilities that companies require.
Efficiency with a human touch
The aim is to devise route plans that are not just short, cost-effective, or fast, but are effective for drivers. Many drivers receive a route plan in the morning and make their own adjustments because they know better than any algorithm where to park safely, how to avoid traffic at certain times of the day, or during which times of the day a customer is more likely to be available. This tacit knowledge ingrained in every driver is impossible to encode in a static implementation of an optimization algorithm or a set of mathematical equations. However, we may be able to come up with more reliable routes by observing the actual behavior of drivers over time. A learning-based approach may be able to capture the tacit knowledge of the most experienced drivers by detecting patterns in the way they execute their routes. A learned routing policy could also be continuously retrained on new data from real-world operations, allowing the model to adapt to changes in driver behavior, for instance, due to construction-related road closures or changes in customer preferences. This is the kind of approach to learning-based route planning we should explore further.
While a few years ago companies treated customers as a uniform mass, now, businesses are taking into account individual requests and characteristics. As a result, they have to capture more stop-specific information, which depends on factors as specific as the nature of the customer’s building. A few years ago, organizations offered time windows, and customers could choose when they wanted to receive their package. Nowadays, the expectation is that the logistics process should anticipate each person’s availability and preferences. Likewise, as high-end logistics services such as same-day delivery are becoming increasingly commonplace, they also need to become more accessible to a broader part of the population, including people who do not live in major urban centers.
High-end logistics services such as same-day delivery must be more accessible to the entire population
The level of service quality and speed we see being provided by state-of-the-art logistics systems would have been unimaginable a couple of years ago. Besides quality and speed, another area of improvement that might be less obvious is sustainability. The logistics and transportation industry is one of the main contributors to carbon emissions and, consequently, climate change. If we do not decarbonize this industry, we will not be able to prevent the worst-case scenario. Without the new methods and innovations we aim to develop in the Intelligent Logistics Systems Lab at MIT, our industry will likely fail to meet the ambitious decarbonization targets that would be required to make a difference.
Research using hybrid methods
Moving forward, we see vast potential in the use of hybrid methods that combine the best of both worlds — connecting traditional OR approaches with ML and AI techniques. To reap this potential, we intend to bundle our research activities on such hybrid methods and their applications in the newly created Intelligent Logistics Lab at MIT. Our goal is to envision the future generation of logistics systems, enabling services that today we can only imagine but will become a reality within the coming years.
The latest trends require us to build systems that are smarter and more agile in responding to changes in the operational, socioeconomic, and geopolitical environments. In Mecalux, we have found a partner who truly believes in these methods. We must learn from the rich knowledge and experience that resides within the many people that run our industry day by day, be it the driver, the warehouse worker, or the supply chain logistics executive with 40 years of industry experience. We need to expand that expertise and ingrain it in quantitative models to solve the ever-more challenging problems faced by our industry both rapidly and at scale.
References:
Dantzig, G. B., and J. H. Ramser. 1959. The Truck Dispatching Problem. Management Science 6 (1): 80–91.