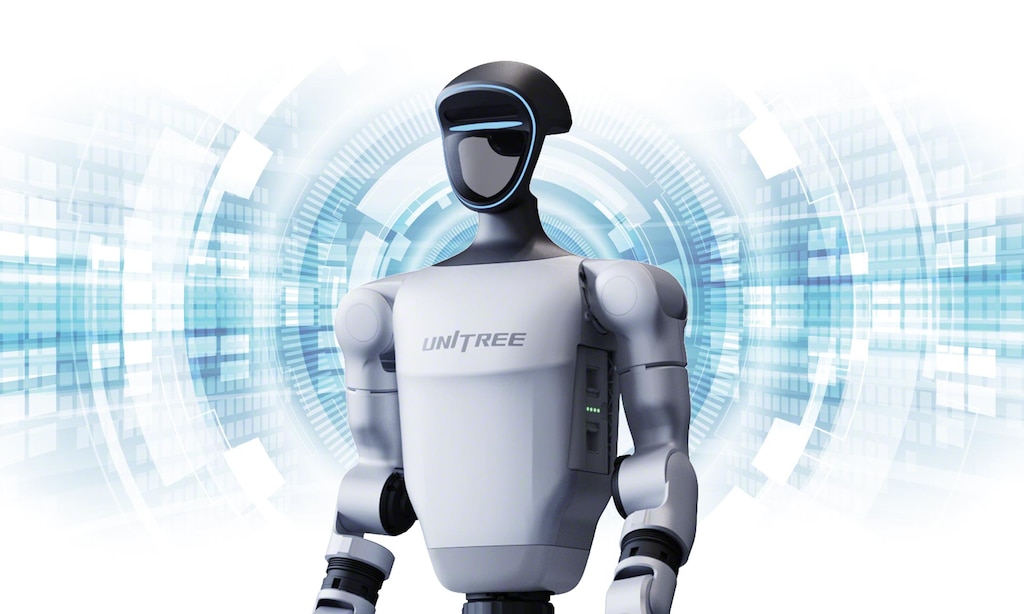
LOGISTICS RESEARCH
By Jay Huang, PhD, Dien Wang, PhD, and Weibin Liang, PhD
Photo: Unitree
Humanoid robots, the ultimate form of robots that mankind has long dreamed of, seem to be on the verge of becoming a reality. Public interest has surged, with global tech giants expressing increasing enthusiasm. However, the industry remains in the prototype stage.
Inspired by human anatomy, humanoid robots’ most fundamental three elements are the brain, for task and motion planning; the cerebellum, for maintaining balance and smooth movement; and the body, for perception and execution. The primary challenges for these robots are preventing falls and enhancing their intelligence to perform generalized tasks.
Artificial intelligence (AI) is the game changer. Emerging AI techniques (e.g., reinforced and imitation learning, large language models) are paving a promising path for humanoid robots. Thanks to the cerebellum, robots can learn how to keep balance, reject disturbance, execute complex movements in virtual worlds, and then migrate these skills to the real world. The AI-enhanced cerebellum shows remarkable advancements in robots’ stability and adaptability, which were formidable hurdles in the past. While the cerebellum is vital, the brain is even more important for humanoid robots. In the near term, reinforcement and imitation learning hold substantial promise for trained applications. The end-to-end large model solution is appealing, but practical implementation in the immediate future remains challenging. Human teleoperation (where a human operator remotely controls a robot) stands out as a practical approach for collecting training data. It could serve as a backup plan if the robotic brain is not well-qualified in the mid-term.
Before adopting AI in this field, androids fell over easily
Regarding the companies developing humanoid robots and their latest progress, Unitree is dominant in terms of the body and the cerebellum. The company has a strong focus on four-legged robots and seamlessly transitioned into the realm of two-legged machines. Its humanoid robot showcased exceptional motion capabilities. On the other hand, Google DeepMind has advanced in robotic brain development. The business is exploring diverse generative AI techniques beyond traditional large models, and its research has been based on a simplified humanoid robot.
The three most crucial elements for humanoid robots are the brain, the cerebellum, and the body (including the eyes, ears, skin, muscles, and bones). The major challenges for the three are relatively independent and can be improved in parallel.
Photo: Unitree
The cerebellum
As with humans, a humanoid robot’s cerebellum plays an essential role in coordinating joint motions to maintain balance and ensure smooth movement. For these robots, walking stably posed a considerable obstacle in the past. Prior to adopting AI in this field, androids easily fell over, necessitating the use of safety cables to protect the fragile machines.
Some players have implemented relatively advanced control methods — e.g., whole-body control and model predictive control — showcasing impressive results in demonstrations. However, humanoid robots have not been qualified to handle unpredictable environmental changes effectively. These machines require further optimization for specific locomotion or tasks, with the cerebellum representing a persistent challenge within the field.
Fortunately, AI (i.e., reinforcement learning) coupled with powerful simulation techniques can be the game changer today. Reinforcement learning is a trial-and-error process: robots can learn behaviors from repeated interactions with the surrounding environment. Engineers program these machines with “what to do” instead of “how to do,” and they will then find ways to complete the tasks on their own.
3D vision using LiDAR or depth cameras, dexterous hands and force/tactile sensing are paramount for humanoid performance
Fine-tuning these movements requires support from simulation, which is a virtual environment for robots to learn the locomotion and tasks. The motion, skills, and knowledge acquired in the virtual simulation can subsequently be transferred to the real world via simulation-to-reality (Sim2Real) frameworks. Failures are inevitable in the initial stages, but eventually, they can find a path to success.
While the future looks promising, technical stumbling blocks exist in simulation environments and Sim2Real. These complex, intricate platforms aim to replicate physical phenomena accurately, including rigid body dynamics, collision, friction, and deformation to create a realistic virtual world.
Boston Dynamics achieved a notable decrease in fall-down frequency — to one time per 31 miles — for its four-legged robot. This number is much higher for two-legged machines. Therefore, despite the strides made in this field, the stability and reliability of humanoid robots should be further polished before widespread deployment in industry.
Tesla Optimus Gen-2 humanoid robot by Tesla
Published under CC BY 3.0 Unported licence
The brain
Researchers are still exploring how to make robots smart enough to carry out generalized tasks. The industry is trying to address the near-term issues via reinforcement and imitation learning. It looks forward to deploying multiple large models and an integrated end-to-end large model in the longer term.
Besides enhancing robots’ motion capabilities, reinforcement learning can be employed to train for specific tasks. However, using it to cover all generalized actions will make the training time too long. A more practical approach involves integrating imitation learning, where a person demonstrates tasks through teleoperation and the robot regards the human behavior as an optimized solution.
Google DeepMind’s team proposed adopting multiple large models to handle the functions of perception, planning, and actuation. The team also introduced an integrated vision-language-action model — Robotic Transformer 2 (RT-2) — to handle all three core functions. It demonstrated that RT-2 can perform generalized tasks that require reasoning, symbol understanding, and human recognition. For example, the task “put the strawberry into the correct bowl” requires the robot not just to understand what the strawberry and the bowl are but also to discern that the strawberry should be grouped with similar fruits.
The body
Beyond the prominent components such as motors and reducers, we believe the following elements are paramount for humanoid performance: 3D vision using LiDAR or depth cameras, dexterous hands, and force/tactile sensing. We expect these elements to be indispensable for the optimal functionality of advanced humanoid robots.
Emerging trends and challenges of humanoid robots
Key humanoid robot modules | Technology trends | Major challenges |
---|---|---|
Brain Task & movement planning |
|
|
Cerebellum Coordination of joint motion |
|
|
Body Perception of environment + motion execution |
|
|
Source: Chinese Academy of Sciences, Beijing Humanoid Robot Innovation Center, Tsinghua University, Bernstein analysis
*Sim2Real, i.e., simulation to reality, refers to transferring motion, skills, or knowledge from a virtual simulation to the real world.
Implications for industry
In the current era of “the robot renaissance,” AI is commonly embedded with vision for tasks involving location, identification, and inspection. However, industrial robots still lack advanced intelligence. Emerging AI technologies (reinforcement and imitation learning, large language models, etc.) may revolutionize the field.
Scientists have demonstrated the feasibility of merging AI with industrial robots in aspects such as the optimization of industrial robots’ movement trajectories and execution time, self-generating strategies for handling complex scenarios, and the simplification of robotic programming processes. Considering the growing maturity of the industrial robot ecosystem, we expect emerging AI to drive further adoption of these machines in the foreseeable future.
AUTHORS OF THE RESEARCH:
Jay Huang, Ph.D, Dien Wang, Ph.D, and Weibin Liang, Ph.D, Research Analysts of Sanford C. Bernstein (Hong Kong) Limited, part of Société Générale Group.
Original publication:
Huang, Jay, Wang, Dien, Liang, Weibin. 2024. “Global Automation: The Humanoid Primer”. Bernstein Société Générale Group.
Please note that this article has been prepared for institutional and professional investors only and is not intended for retail or private investors. Please visit www.bernsteinresearch.com for important disclosures.