
GenAI and its potential in the supply chain
GenAI and other branches of artificial intelligence are being increasingly adopted by companies striving to remain competitive. But what exactly does this term mean?
What is GenAI?
GenAI — generative artificial intelligence — focuses on developing algorithms and models capable of creating new content, including images, texts, or routes. Beyond analyzing preexisting data, it can generate original ideas and solutions to a wide range of problems. To do so, it employs advanced machine learning techniques such as deep learning.
While AI traces back to the 1950s, GenAI has become what it is today thanks to several 21st-century milestones. Towards the end of the 20th century, in 1997, Deep Blue demonstrated AI’s ability to solve complex problems by defeating world chess champion Garry Kasparov. The 2010s then witnessed the deep learning revolution. This transformed AI’s capabilities in speech and image recognition and gave rise to new generative models.
As a result, GenAI can classify and categorize information, analyze and adjust strategies and plans based on real-time data, generate multiple pieces of content automatically and simultaneously, summarize large texts by extracting key details, and provide instant responses in voice or text format. GenAI also has applications in the supply chain.
How can generative AI in the supply chain drive value?
Applying generative AI to the supply chain can benefit various areas and processes, from the beginning stages to last-mile delivery. According to EY, 40% of supply chain organizations are investing in GenAI, which has multiple applications in the following areas:
Planning
Through GenAI, companies can create scenarios to simulate outcomes and evaluate several action plans before implementing any initiatives. This technology is valuable for demand forecasting, production planning, and proactively managing potential risks.
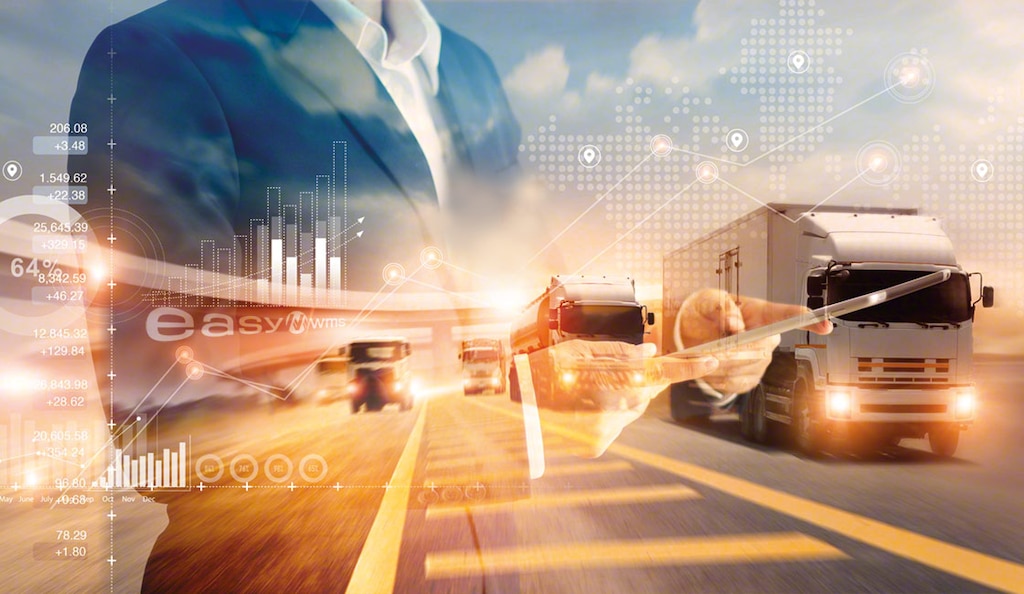
Procurement
Employing GenAI to analyze information enables businesses to negotiate costs and purchase products and raw materials more quickly. Similarly, with this tool, they can monitor and analyze supplier performance, streamline their selection, and obtain recommendations and rankings before making a decision. Another area where GenAI proves helpful is contract renewals.
Manufacturing
GenAI also has applications in fields such as predictive maintenance, where it can identify machines or production lines that might fail in the coming hours or days. This branch of AI could expedite the product design and marketing phases.
Distribution
One of the clearest applications of GenAI in the supply chain is the optimization of operator pick paths and vehicle delivery routes. For instance, distribution can be customized based on criteria such as fuel consumption, shipment priority, or traffic. GenAI can also use historical data or weather information for this purpose. This leads to shorter delivery times, reduced costs, and improved customer service.
GenAI in logistics
In addition to the functions mentioned above, GenAI can be an ally in these everyday logistics tasks:
- Inventory optimization. GenAI allows companies to adjust their inventory levels by analyzing past demand and forecasting future requests. This enables them to achieve optimal values, avoiding shortages and overstock.
- Customer experience personalization. GenAI systems can adapt to the preferences of each user, recommend related products, and offer real-time parcel tracking.
- Logistics scenario simulation. Studying the impact of scenarios such as adverse weather conditions or changes in available resources allows organizations to anticipate potential issues. This is a prime example of GenAI in action.
- Detection of supply chain anomalies. GenAI models can spot situations such as unusual delivery delays and demand fluctuations. This helps businesses prepare for these scenarios, making GenAI a strategic asset for the supply chain.
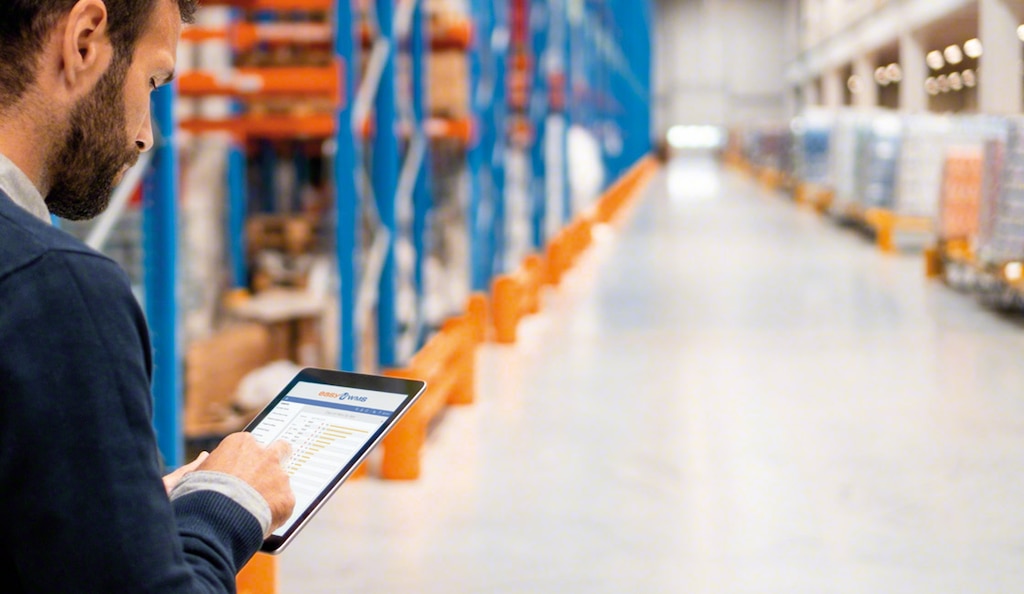
GenAI vs. artificial intelligence
AI systems, whether local (edge AI) or cloud-based, are used for analysis and predictions. GenAI, however, goes a step further by creating information similar to what it was initially trained on. It moves beyond simple processing to generate new, unique content. The main difference between the two technologies lies in the complexity and objectives of their algorithms.
AI | GenAI | |
Objetives | Data analysis, process automation, and decision-making based on existing information. | Creation of new, original content. Generation of information and ideas. |
Characteristics | Pattern recognition, predictive modeling, and decision trees. | Deep learning, neural networks, and data generation. |
Use of data | Relies on structured data for specific tasks. | Employs both structured and unstructured data. |
Applications | Predictive analytics, recommendations, automation. | Automated creation, data generation, content moderation. |
Technological approach | Structured analysis and logical processes. | Dynamic, creative, and adaptable processes that provide innovative results. |
Effects on industry | Wide range of applications in specific rule-based tasks across various sectors. | Transformational capability in data synthesis and content generation. |
Improve your logistics operations with GenAI
Looking to leverage the latest technologies to boost your business? You’ve come to the right place. At Interlake Mecalux, we specialize in automated solutions and their supervision with our Easy WMS warehouse management system. Additionally, we enhance visibility for large distribution networks through Easy DOM. With extensive experience in intralogistics since 1966, we explore the potential of GenAI in software programming and development. Be sure to contact us. Together, we’ll take your logistics processes to the next level.