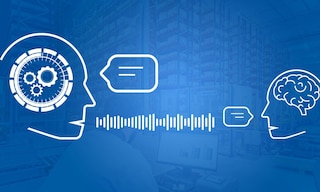
Conversational AI in industry and logistics
As artificial intelligence (AI) continues to advance, it becomes capable of simulating more and more processes that used to rely on direct human intervention. Conversational AI — along with other trends such as computer vision — is already being employed by companies across various applications. Moreover, it’s incorporated in numerous devices found in industries, offices, and homes.
What is conversational AI?
Conversational AI is artificial intelligence trained to simulate human conversation. To accomplish this, it utilizes natural language processing (NLP)— a subset of AI that enables computers to understand and process communication between people — large volumes of data, and machine learning technologies. To understand exactly what conversational technology is, consider chatbots. These virtual agents are programmed to assist users, answering their questions and scheduling appointments. But these aren’t conversational AI’s only capabilities.
What technology does conversational AI leverage?
This intelligence needs to be trained on large volumes of data — whether speech or text — to understand and process different languages. To do so, conversational AI employs a combination of NLP, foundation models, and machine learning.
- Machine learning. This field of AI comprises algorithms and data that continuously improve through experience. AI enhances its ability to recognize patterns, applying this to make more accurate predictions.
- NLP. This method is used to analyze language — with the help of machine learning — in conversational AI. Additionally, machine learning algorithms enhance the quality of dialogues as the NLP system learns. It consists of four steps that transform unstructured data into a computer-legible format for the machine to generate an appropriate response:
- Input generation. Users enter information in speech or text format into a webpage or app.
- Analysis. For text-based input, conversational AI employs natural language understanding (NLU) to decipher its meaning and intent. If the data are speech-based, it will combine NLU with automatic speech recognition (ASR).
- Dialogue management. Next, natural language generation (NLG), a subset of NLP, formulates a response.
- Reinforcement learning. Lastly, machine learning algorithms refine the information over time to optimize quality.
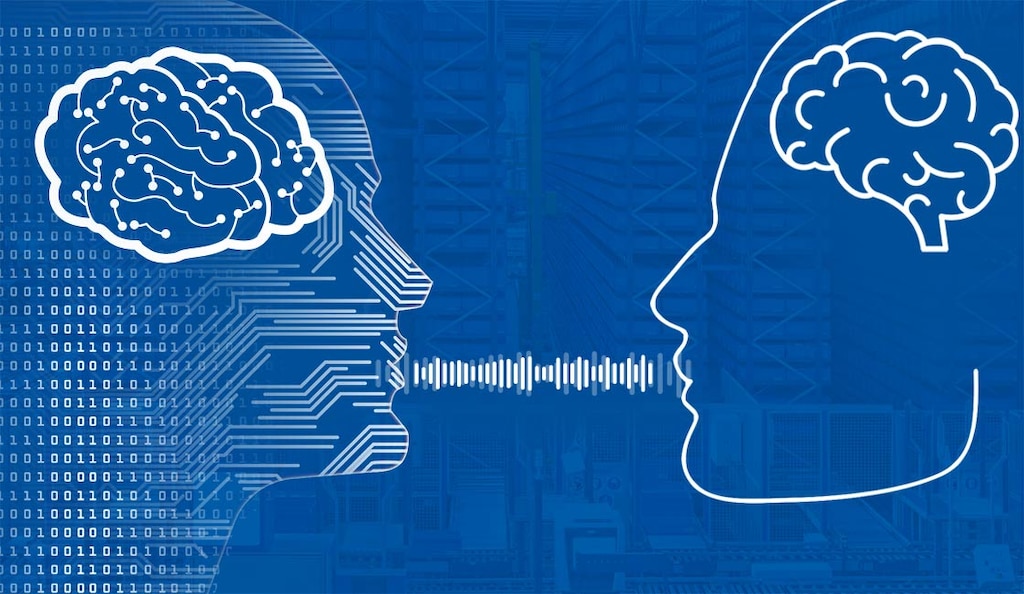
Conversational AI examples
Conversational AI technology is present in numerous apps under various names:
- Generative AI. This technology leverages conversational AI to improve oral and written exchanges.
- Chatbots. Typically used in customer service, these bots respond to frequently asked questions and offer assistance.
- Virtual assistance. Generally voice-activated, these agents are compatible with mobile devices and smart speakers.
- Text-to-speech software. This is employed to create audiobooks and provide instructions, such as those from screen readers often used by individuals with partial or complete blindness.
- Speech recognition programs. These apps transcribe conferences or phone calls. They can also generate subtitles in real time.
Thus, conversational AI has become a valuable tool for accessibility, online customer service, devices operating in the Industrial IoT (Internet of Things), and even home speakers. It’s also found in business intelligence apps. Conversational business intelligence combines conversational AI with data analysis capabilities, enabling users to visualize and obtain explanations of results.
Advantages of conversational AI for business
Leveraging AI trained to simulate dialogue offers several benefits:
- 24-hour customer service. Conversational AI assists people at any time, without the need for human agents nearby.
- Cost savings. This type of support requires fewer resources to provide customer service, for instance.
- Task automation. Implementing this technology for tasks such as text transcription can reduce human errors and speed up processes.
- Personalized experiences. These systems remember customers’ preferences and history and are increasingly employed in omnichannel strategies. This processing helps to boost sales and enhances users' perception of a brand.
- Scalability. Conversational AI’s capabilities can be expanded rapidly, making it an asset for demand peaks like Black Friday.
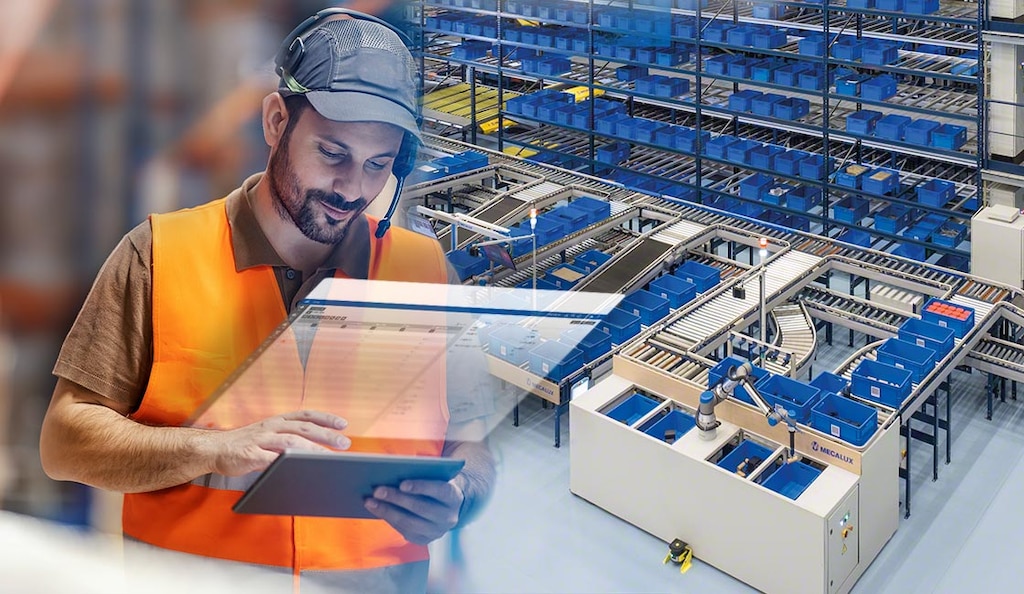
Conversational AI design
Several tools can be utilized to build chatbots and conversational AI technologies, but their design still calls for human intervention. Customer service models are trained on machine learning as well as real-life conversations. This speech is labeled by human analysts and call center agents. It’s further enriched with data on user behavior (e.g., previously visited webpages), order status, and even external sources like weather and potential events. This approach enables smarter predictions and faster troubleshooting. Machine learning is also used to scan dialogues between customers and operators to determine patterns.
Difference between a chatbot and conversational AI
The line between chatbots and conversational AI may seem blurry. However, the former is essentially derived from the latter. Conversational AI encompasses a set of technologies crucial for developing chatbots. In other words, an intelligent chatbot is an app based on a conversational AI platform.
While a chatbot is a program that simulates conversations, conversational AI enables omnichannel user interfaces, provides context, processes language, anticipates customer needs, and integrates with other systems featuring advanced analytics. A chatbot is less complex.
AI: an asset for your warehouse
At Interlake Mecalux, our mission is to provide our clients with cutting-edge logistics facilities that leverage the latest advances. These include AI-driven picking operations and stacker crane positioning. As automation experts, we developed Easy WMS, our proprietary software designed to raise warehouse throughput. Feel free to contact us for advice on this and other warehousing solutions.