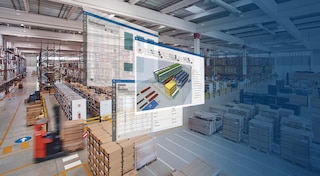
Predictive analytics: telling the future
We live in a world in which all actions we take in our social and work lives constantly and automatically generate mass data. In this context, predictive analytics converts these data into valuable information that allows us to anticipate and predict future scenarios, which is essential nowadays for Logistics 4.0.
It’s not enough to merely collect data; what makes the difference are companies that know how to transform those data into useful information. According to the Workforce 2020 report from Oxford Economics, this is something only 42% of businesses know how to do.
Applying predictive analysis to logistics makes operations more efficient and more accurate, as well as drastically reducing associated costs. Want to know how to do this? We’ll tell you how in this post.
What’s predictive analytics?
The concept of predictive analytics refers to a type of analysis that uses both historical and real-time data to predict behaviors, trends, and habits in individuals and machine performance.
As the predictions of these scenarios are highly reliable, organizations can make the best business decisions. For example, thanks to predictive analytics, companies can preempt customer demands based on controllable factors, such as sales price, and external factors, such as work calendars and weather effects.
Between 1980 and 1990, banks and insurance companies were the first to implement the predictive analytics technique of data mining. Over the years, predictive analytics has benefited from the emergence of big data systems, IoT (Internet of Things) devices, and the fact that businesses gather more and more historical data to process in real time. This has increased the opportunities for analyzing and transforming those data into predictive information.
Differences between descriptive, predictive, and prescriptive analytics
To better understand the concept of predictive analytics, it’s a good idea to differentiate between the various types of data analysis:
- Descriptive analytics: this uses historical data to take a snapshot of how the business is performing to date. It can quantify, reflect, and analyze what has occurred.
- Predictive analytics: this aims to anticipate or predict what could happen based on historical data. Its purpose is to make forecasts and stay ahead of what is slated to happen.
- Prescriptive analytics: this describes what to do to ensure that the firm’s forecasts come to fruition or how to prevent them in the event that they are negative. It uses simulation and optimization techniques to define the best path to follow.
Broadly speaking, the objective of this analysis is to collect all possible data and convert them into the information a company needs to understand what has occurred, what will happen, and what can be done to guarantee (or avoid) this.
Predictive analytics: advantages
Predictive analytics and better decision making offer a number of benefits that will help you to strengthen your business. In the logistics sector, we can optimize the following competencies:
- Demand analysis. Data linked to sales history and the market are gathered to analyze aspects on potential consumers to, thus, know what it is they want.
- Inventory management. Through predictive analysis, you can more accurately predict the amount of goods you need to establish safety stock and minimum stock levels in addition to avoiding stockouts.
- Replenishment planning. By being able to anticipate demand, you can efficiently manage a product’s reorder point, ensuring availability both in the warehouse and at points of sale, thereby improving customer satisfaction.
- Resource optimization. The information obtained is employed to better leverage available resources, both human and in terms of infrastructure.
- Cost savings. Predictive analysis cuts operating costs, whether fixed or variable.
The data forecasting produced by predictive analytics enables you to more accurately anticipate future activity, behavior, and the most appropriate logistics and supply chain KPIs for making decisions that bolster your business.
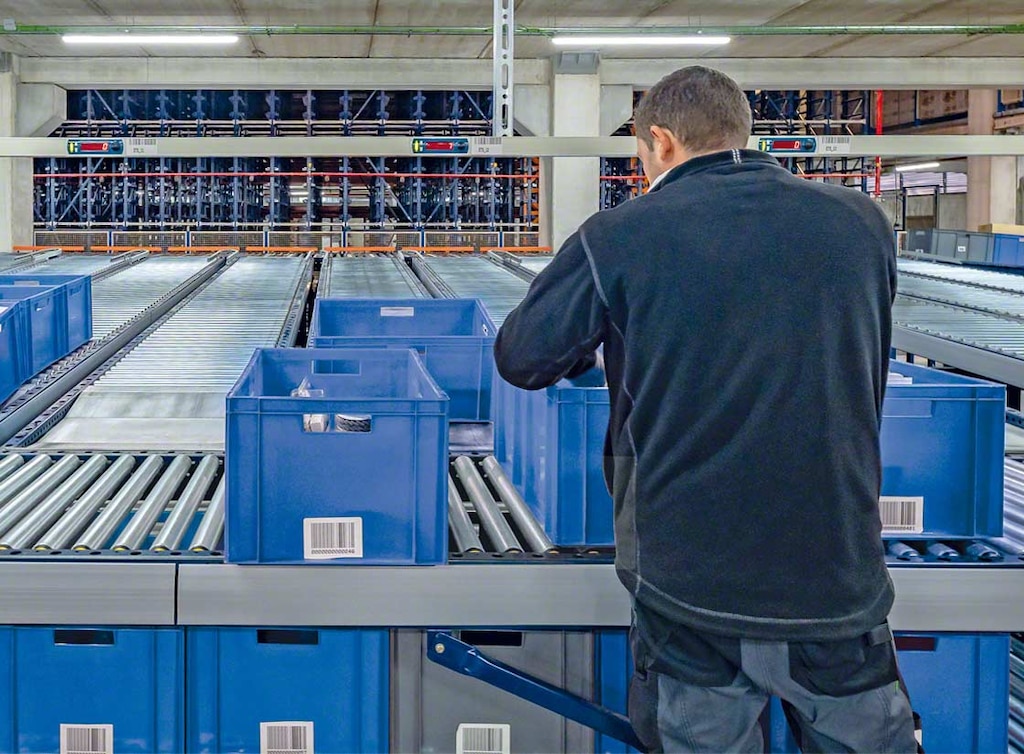
Predictive analytics algorithms and models using big data
Predictive analytics applies advanced mathematics, statistical analysis, and automated algorithms to find patterns in the data collected via big data technology.
Once you’ve defined your objectives and selected the data to be processed, it’s time to work with the algorithms that will help you to know in advance what will happen. The algorithms that play a part in predictive analytics can be classified into three major types:
- Clustering algorithms: these are applied to segment customers in order to target a marketing campaign, define the duration of an offer or promotion, etc.
- Classification algorithms: these are charged with building customer loyalty through increased knowledge of their habits and behaviors. It lets you design personalized offers, improve service, and detect whether the customer intends to buy more products.
- Regression algorithms: these are focused on providing reliable forecasts based on objective data with the aim of, for example, optimizing stock management and predicting increased demand for a product.
So, to recap, there are various types of algorithms, each of which fulfills different functions.
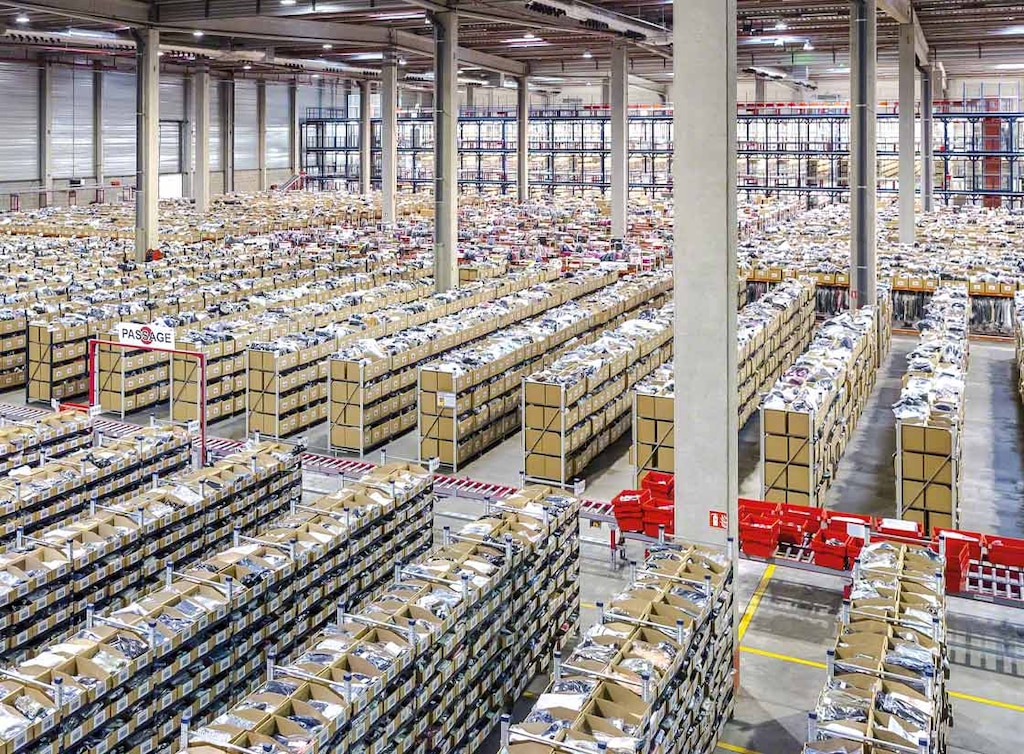
Predictive analysis tools
Various tools can be employed to get the most out of predictive analytics in your business model and, more specifically, in your supply chain:
- Excel: although its purpose isn’t to collect data, it can be employed to dump the data collected or that have been manually entered into the Excel table to structure all that information for analysis.
- WMS: warehouse management systems, such as Easy WMS, continually manage activity being carried out in an installation. They turn that information into data that predict a possible scenario in order to make better decisions.
- General tools: tech giants such as IBM and Microsoft, among others, have predictive analytics tools that help companies to gain customer loyalty, boost their income, and determine the right time for changing suppliers. For instance, IBM offers predictive analytics solutions that deduce the level of customer satisfaction based on the information gathered.
It’s important to take into account that, although predictive analytics tools provide extremely valuable knowledge and information, they don’t directly automate decision making.
Example of predictive analytics in logistics
The use of predictive analytics in logistics is primarily centered on predicting the demand that a business’s products or services will have. For instance, predictive analytics is extremely valuable for logistics providers, as it enables them to foresee future needs and, thus, optimize the management of two key aspects: fleet planning and demand in the warehouse. The objective? To maximize available resources to reach the productivity targets agreed to with each customer.
An example of this strategy in the food sector is the Danone warehouse in Madrid, Spain. The multinational has implemented the Supply Chain Analytics module, which will serve to check and analyze all the data generated by Easy WMS. This app transforms data into relevant information to reflect what’s happening in the facility and enable the firm to make strategic decisions for improvement as well as for anticipating future scenarios.
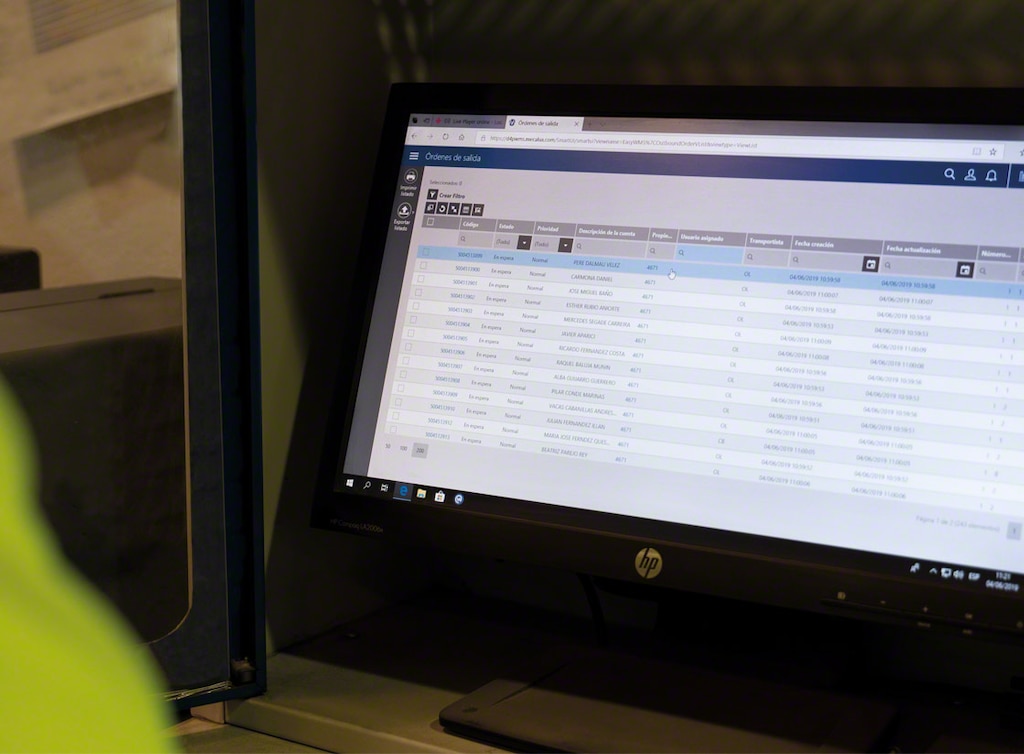
Data mining for staying one step ahead
Predictive analysis is a continuously growing tool. It allows companies not only to make better decisions, but also to assess their current business model to see whether an overhaul is in order to meet future needs.
Storage installations are an infinite source of data. With the incorporation of a warehouse management system, you can transform them into very valuable information to bolster your business decisions. If you haven’t yet installed a WMS, get in touch with Interlake Mecalux. We’ll advise you on how to get the most out of the technologies revolutionizing the supply chain, such as predictive analytics, big data, and machine learning.